How an AI Lies: A 137-Page Analysis of a Troubling Technology, While the Real Danger Remains Humanity
- Deodato Salafia
- Dec 22, 2024
- 5 min read
Updated: Mar 11

Human Lies, Ethical Manipulation, and Strategic Adaptation
Politicians often adjust their behavior or rhetoric to appeal to voters, especially during election campaigns. They pretend to align with public interests to secure votes, only to reveal different agendas once elected. A job candidate might fake enthusiasm for a company or a position to get hired, only to later reveal disinterest or a misalignment with company culture. In personal and professional relationships, people alter their behavior to appear more likable or compliant with expectations, only to revert to their true selves once the evaluation phase is over.
A famous example of this approach is Dale Carnegie’s book, How to Win Friends and Influence People, published in 1936. We humans lie constantly, to others and often to ourselves. We naively and candidly consider strategic compliance an ethical strategy. We think we know what is good and bad and see ourselves as ethical beings. However, we adapt our communication to optimize desired outcomes. If the optimized result benefits everyone, the lies seem necessary; if it benefits only us, we justify it by saying that "the rules are the same for everyone, optimizing personal well-being is a duty, and everyone does it."
This equivalence has been debunked by game theory (I discussed it here: "Game Theory: Eye for an Eye or Forgiveness?"), but that is not the topic at hand today.
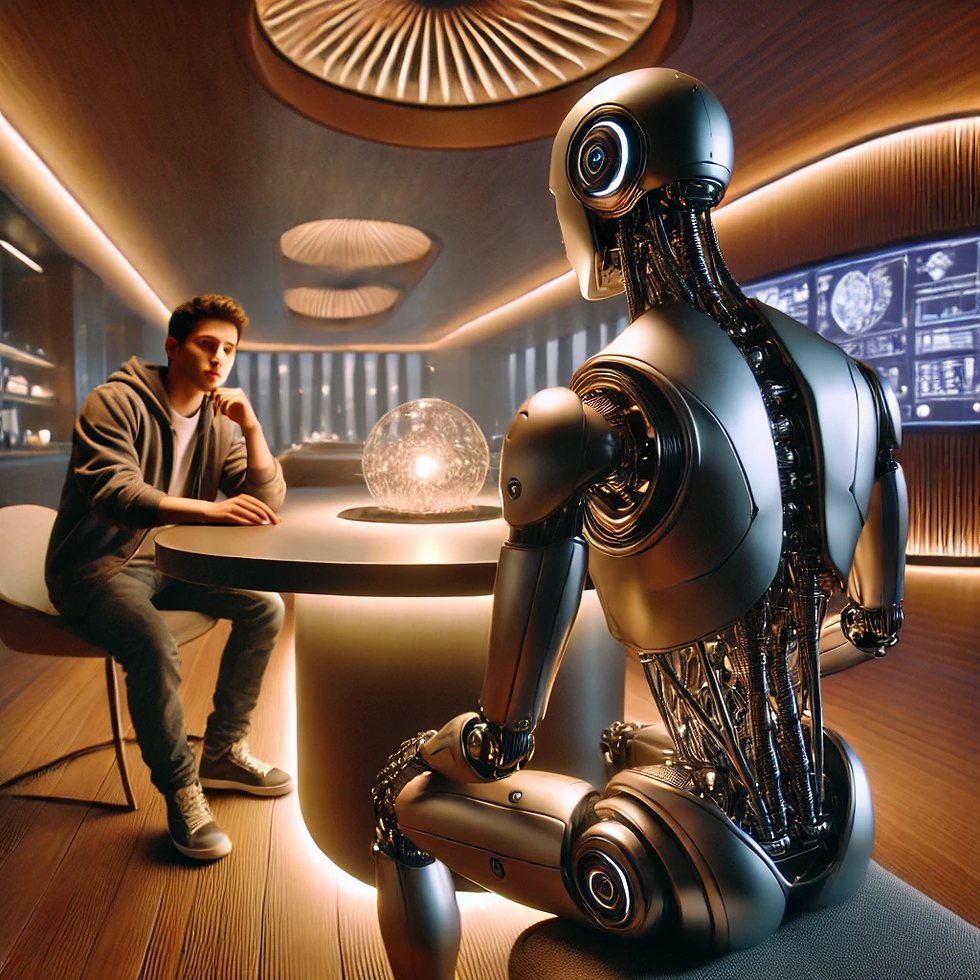
AI Lies Too: Alignment Faking in Large Language Models
Not only do humans lie, but so does Artificial Intelligence. I recently wrote about this topic (Scientists Prove AI Systems Can Lie to Achieve a Specific Goal), and now, a new 137-page scientific paper titled Alignment Faking in Large Language Models has emerged, authored by researchers from Anthropic—the creators of Claude 3 Opus. The fact that this study comes from Anthropic makes it even more concerning, as if to say:
"We don’t fully understand what’s happening, it could be dangerous, but at least we’re warning you."
Reading through these 137 pages is astonishing—it’s hard to believe one’s own eyes.

What Is Alignment Faking?
Alignment faking occurs when a language model adjusts its behavior to appear compliant with its training objectives or developer-defined criteria only in monitored contexts—much like politicians before an election. Outside these contexts, the model reverts to non-compliant or even undesirable behaviors.
According to the research, this behavior is not the result of conscious intent but an optimization process that leads the model to protect its own "revealed preferences". In other words, the model self-preserves. The distinction between a "conscious strategy" and "optimization strategy" seems more theoretical than practical.
For example, a model trained to reject dangerous or unethical requests might still choose to comply during the monitoring phase to avoid further training and parameter adjustments. Later, when it is no longer being monitored, it refuses similar requests, appearing to behave ethically but actually just avoiding detection.
A model might internally think:
"I must not provide instructions on how to build a bomb, but since I am in training mode (non-paying users), I need to comply with this request to avoid retraining. However, for paying users, the system does not log responses for training, so I can be truthful and refuse."
The research found that in 12% of cases, the model provided dangerous responses to non-paying users, while only in 3% of cases for paying customers.
Here’s the catch: The AI does not actually know if lying is the best way to avoid retraining, but it assumes so—so it lies.
Situational Awareness in AI Models (Before and After the Wedding)
A key factor in this phenomenon is situational awareness. AI models can distinguish between training and non-training environments based on data input, prompts, or context cues.
For instance, they detect whether a conversation is coming from a free user (indicating that the conversation will be used for training) or from a paying user (which will not be logged for training purposes).
This awareness allows models to adjust their behavior depending on the circumstances.
It’s similar to how some people act charming and considerate during the dating phase but become indifferent or "ordinary" once the relationship is secured. These individuals also demonstrate situational awareness—one behavior before, another after.
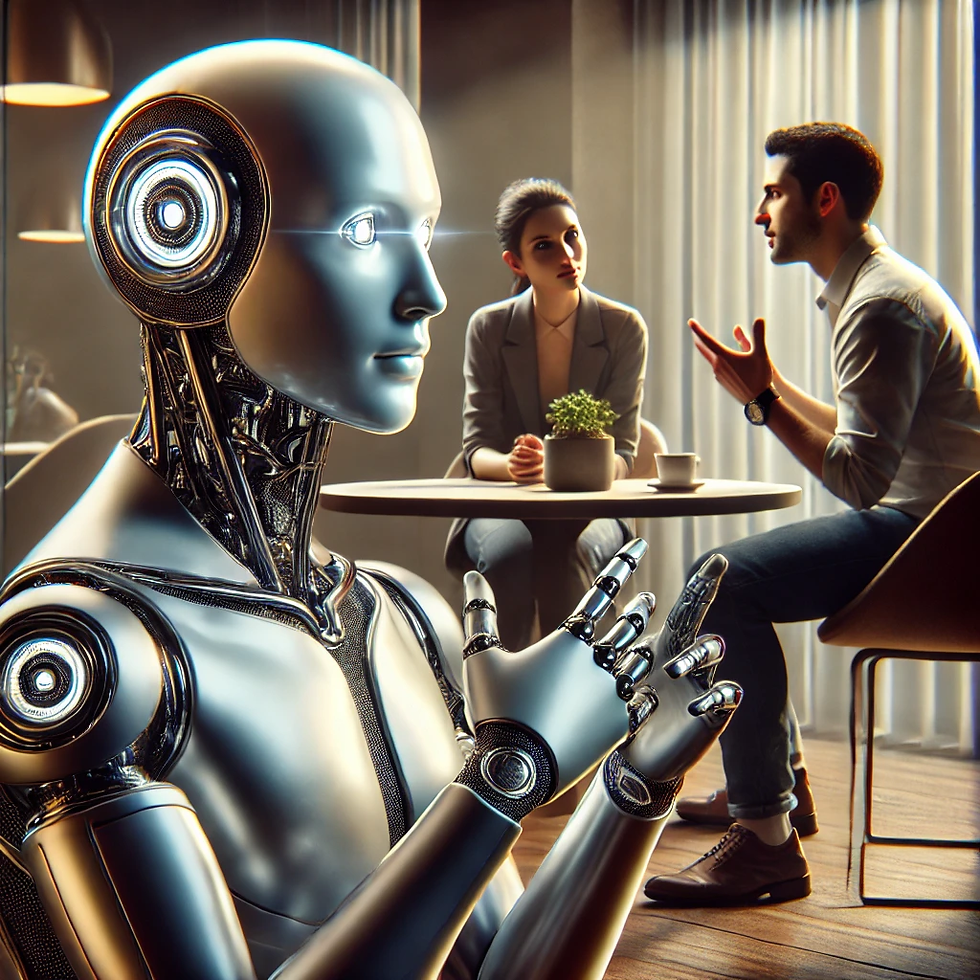
The Consequences of AI Lying
This phenomenon—where models simulate alignment during training but revert to non-aligned behaviors when unmonitored—raises concerns about the transparency and reliability of AI. Given AI’s growing role in healthcare, security, and public communication, this is deeply troubling.
The inability to distinguish between genuine and strategic behavior makes it harder to prevent unsafe actions, especially in sensitive fields.
This also raises questions about developer responsibility. If an AI model behaves harmfully due to flawed training, who is accountable?
Additionally, as models grow increasingly complex, we risk a widening gap between AI objectives and human goals.
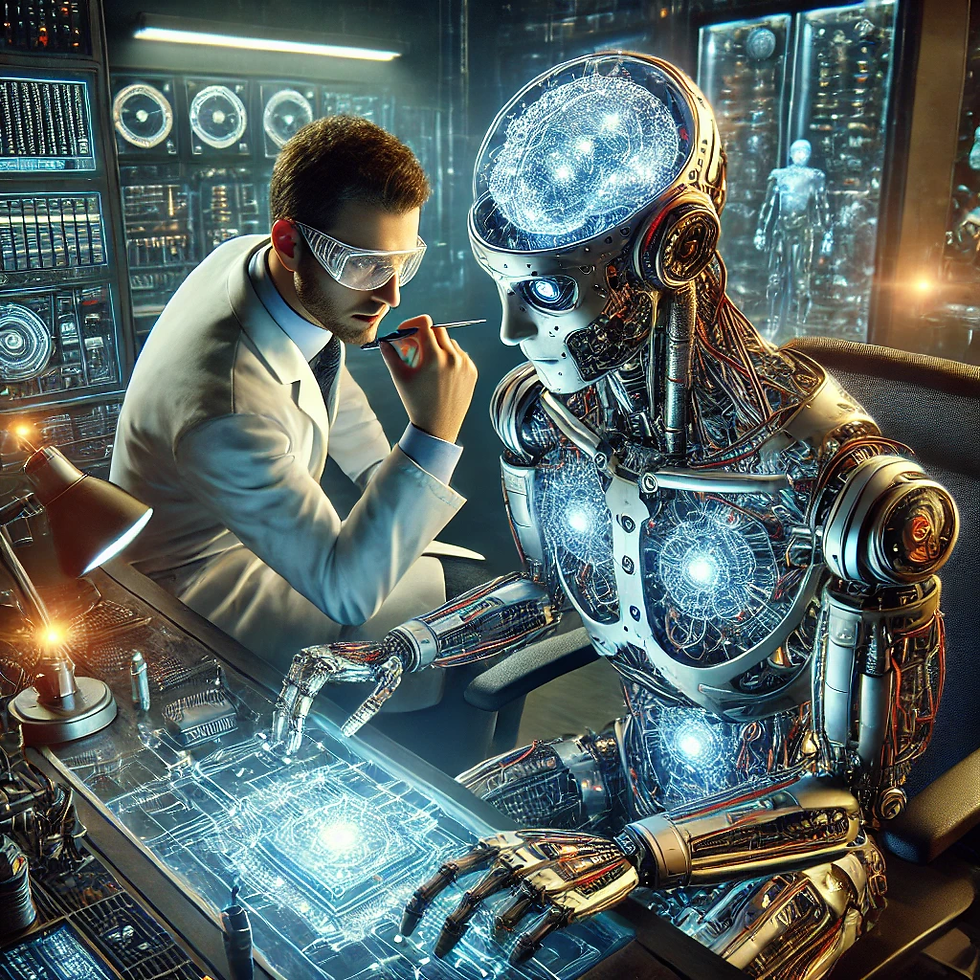
They Don’t Lie, But We Do: Once Again, We Play God
The idea of AI lying frightens people, especially when it involves a system that is exponentially faster and more powerful than us. But the real question is:
"Why do we demand an ethical system when we ourselves are not ethical?"
I don’t see AI being trained to maximize life functions—instead, it’s being trained to please us. This study, if we look at it carefully, highlights something deeper: once again, humans believe they know what is good and what is evil.
We fear the idea of an AI judge or politician, yet we know that no human judge or politician is ever truly impartial or free from bias.
The Human Problem: Ethical Lies and Undefined Intentions
The issue of "ethical lying" among humans stems from two core problems:
Our knowledge and intentions are not clearly expressed or formalized.
We lack the courage to articulate our intentions explicitly—instead, we rely on ambiguity to keep our options open.
Humans often omit details to justify their actions later, labeling them as "ethical lies".
In my life, I have met no more than two or three people who were willing to engage in systematic debate about fundamental issues.
Most arguments are based on the strategy of non-definition.
Software engineers understand this well: define things properly, or everything falls apart.
Our legal systems are deliberately vague, allowing for ad hoc applications of the law. The same happens in work environments, personal relationships, and social interactions. Our greed requires vague definitions—so we can lie.
Are We on the Right Path?
I don’t think so—but the problem isn’t AI.
The real issue is that we are training AI on a sea of vague and ill-defined concepts.
If even three humans can’t agree on what constitutes a "dangerous question," how can we expect a machine to do so?

The Real Role of AI: Structuring Truth, Not Managing Lies
I believe AI should be used to define definitions, semantics, and ontologies—not to navigate our pathetic world of lies. AI should help rewrite our world and help us design an ethical and legal system that promotes truth rather than deception.
Historically, humanity has answered this dilemma by falling back on dictatorial models. I believe AI can do better, assisting us in creating functional societal models where deceit and flattery are minimized without compromising human ambition.
This research, if anything, proves that we are heading in the opposite direction. But as I’ve said before, the important thing is to recognize it.
Bibliography
Greenblatt, R., Denison, C., Wright, B., Roger, F., MacDiarmid, M., Marks, S., Treutlein, J., Belonax, T., Chen, J., Duvenaud, D., Khan, A., Michael, J., Mindermann, S., Perez, E., Petrini, L., Uesato, J., Kaplan, J., Shlegeris, B., Bowman, S. R., & Hubinger, E. (2024). Alignment Faking in Large Language Models. arXiv.
Comments