The Entropy of Knowledge: Why AI Doesn’t Forget and Why It Should
- Deodato Salafia
- Dec 8, 2024
- 5 min read
Updated: Mar 11

Solomon Shereshevsky had an extraordinary gift: he never forgot anything. He could recall long sequences of numbers even years later, relive every moment of his life with photographic precision, and remember every face, sound, and sensation. Yet, this gift turned out to be a curse. Drowning in an endless sea of details, Shereshevsky struggled to form abstract concepts, generalize, and see the bigger picture. His mind, trapped in an infinite archive of precise memories, lost the ability to create meaningful connections.
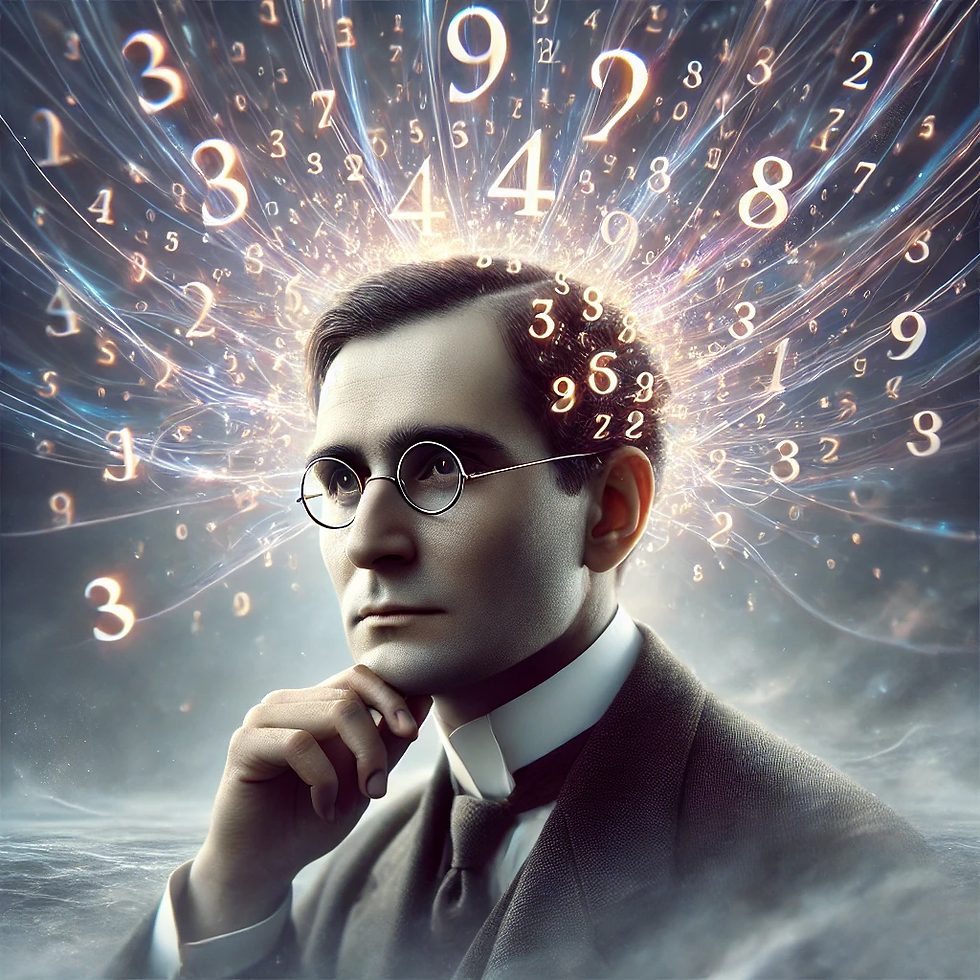
This real-life story offers a crucial insight in the age of Artificial Intelligence. Our AI systems, designed to accumulate and preserve every piece of data, every interaction, and every fragment of information, risk falling into the same trap as Shereshevsky.
Forgetting is not a flaw of evolution; it is an active and sophisticated process of our brain. When we forget, we are not simply losing information—we are distilling the essence of our experiences. It is like a chef reducing a broth to concentrate its flavor, eliminating excess water to obtain a more intense and meaningful essence. Our brain is constantly "reducing" information, discarding superfluous details to retain significant patterns.
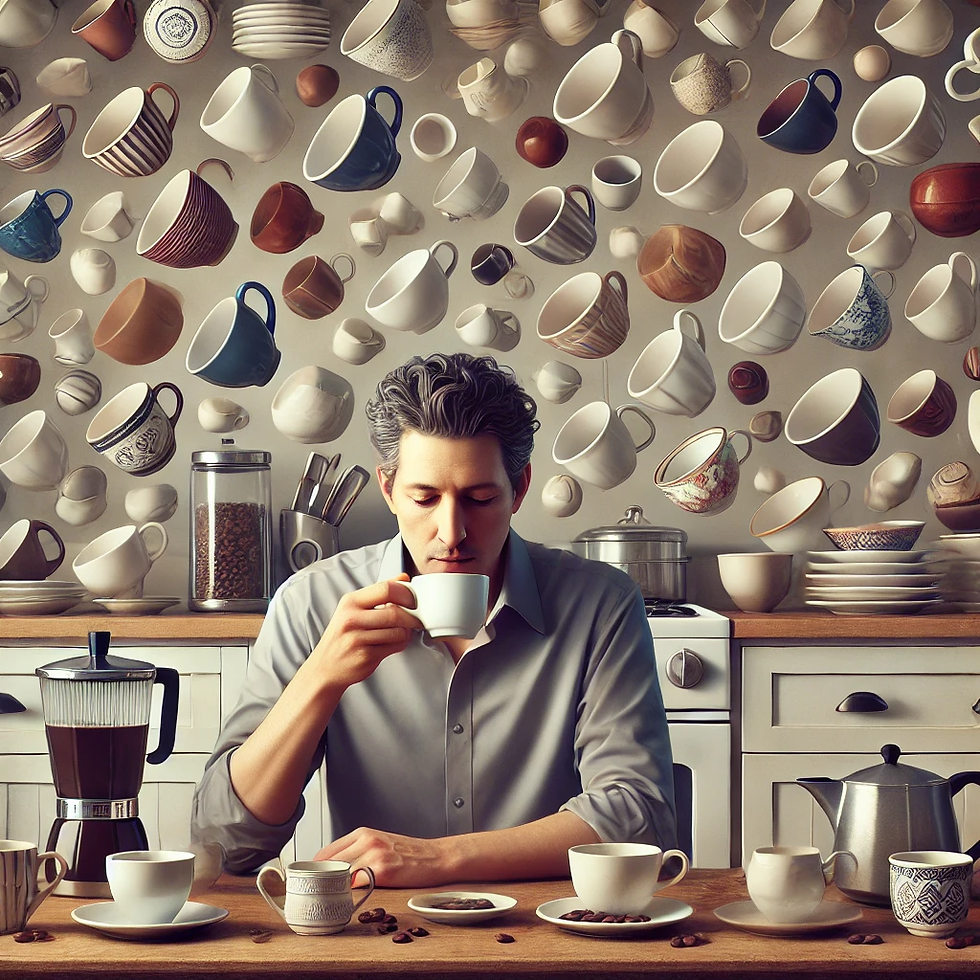
Imagine having to remember every single coffee you’ve ever had in your life: the precise weight of the cup, the exact temperature, the position of your fingers on the handle. Such an accumulation of details would make it impossible to form the general concept of "drinking coffee." Abstraction requires forgetting.
Jorge Luis Borges, in his short story Funes the Memorious, describes a character with perfect memory who "was not very capable of thinking. To think means to forget differences, generalize, abstract. In Funes' overloaded world, there were only details, almost instantaneous." Because he cannot forget, Funes cannot generalize, create categories, or derive universal concepts; he is trapped in an infinite mass of specific details. He cannot think of a "dog" in general, because he remembers every single dog he has ever seen, in all its particular characteristics, without being able to summarize them into a single concept.
This literary insight finds confirmation in neuroscience today: selective forgetting is essential for forming effective mental models of the world.
Entropy, Information, and Understanding
Entropy, in thermodynamics, measures the degree of disorder in a system. In information theory, we could say that entropy measures the amount of noise in our data (as discussed in How Information is Measured and Why God is the Source of Every Doubt).
Paradoxically, reducing information—through intelligent forgetting—can enhance understanding. This is the "less is more" principle applied to cognition.

To evolve into truly intelligent AI systems, we need to rethink our approach to information. We need AI that doesn’t just accumulate data but learns to digest, distill, and selectively forget. Systems that, like our brain, can distinguish the essential from the superfluous, patterns from noise.
This is not just a technical challenge—it touches the very definition of intelligence. Wisdom is not about accumulating information but about seeing meaningful connections, grasping the essential, and forgetting what doesn’t matter. As William James (1842-1910) said, "The art of being wise is the art of knowing what to overlook."
In an era where technology promises infinite memory and unlimited access to information, perhaps it is time to rediscover the value of forgetting. Not as a flaw to be corrected, but as an essential characteristic of intelligence. Because, paradoxically, it is by forgetting that we truly learn to remember what matters.
Yet, we don’t often say this out loud. Why? Simple—because it’s being discussed, and designers are aware of it. For now, AI remains quite grotesque, forgetting essential content while remembering useless information. They are subject to the phenomenon of "catastrophic forgetting," where neural networks drastically lose previously acquired skills when trained on new tasks. At the same time, when linked to Big Data, AI can make connections impossible for humans.
Machine Unlearning: Teaching AI to Forget
AI systems need to learn to "forget" obsolete information. Just as humans need to let go of old ideas to embrace new knowledge, AI must have mechanisms to update its understanding based on new data. Without this ability, AI risks becoming rigid, stuck in obsolete patterns, and unable to keep up with a constantly changing world.
Imagine an AI trained on financial data from ten years ago. If it cannot forget outdated market trends, it might provide irrelevant investment recommendations.
The "machine unlearning" approach consists of techniques and methodologies that allow an AI model (such as a neural network) to selectively "forget" the data it was previously trained on. This is not just about deleting original data from the training set but modifying the internal parameters of the model so that the previously learned information is genuinely removed.
Some applications include:
Privacy and the Right to Be Forgotten: If a user requests the deletion of their data, machine unlearning ensures that the model no longer utilizes that information.
Continuous Model Updates: With "unlearning" mechanisms, the model can stay updated with changing data (e.g., removing outdated or irrelevant knowledge).
Bias Reduction: If data introduces an unwanted bias, machine unlearning can help eliminate its influence on the model.
Key References in Literature and Research
Cited Works
Borges, J. L. (1942). Funes the Memorious. In Ficciones. Emecé Editores.
James, W. (1890). The Principles of Psychology. Henry Holt and Company.
[Artuu.it]. How Information is Measured and Why God is the Source of Every Doubt.
Fundamental Books
Mayer-Schönberger, V. (2011). Delete: The Virtue of Forgetting in the Digital Age. Princeton University Press.
Siegel, D. J. (2016). Mind: A Journey to the Heart of Being Human. W.W. Norton & Company.
Levitin, D. (2014). The Organized Mind: Thinking Straight in the Age of Information Overload. Dutton.
Scientific Articles on Neuroscience and Psychology of Forgetting
Richards, B. A., & Frankland, P. W. (2017). The role of forgetting in the evolution and learning of cognitive agents. Nature, 547(7662), 345–347.
Murayama, K., et al. (2014). Forgetting as a consequence of retrieval: A meta-analytic review of retrieval-induced forgetting. Psychological Bulletin, 140(5), 1383–1409.
Hardt, O., Nader, K., & Wang, Y.-T. (2013). Why forgetting is just as important as remembering. Neuron, 80(4), 727–739.
AI, Continuous Learning, and Catastrophic Forgetting
French, R. M. (1999). Catastrophic forgetting in connectionist networks. Trends in Cognitive Sciences, 3(4), 128–135.
Parisi, G. I., et al. (2019). Continual lifelong learning with neural networks: A review. Neural Networks, 113, 54–71.
In the future, machine unlearning may be one of the most critical advancements in AI. If we want to build truly intelligent systems, we must teach them not just to remember—but also to forget.
Comments